Using estimation models to improve sustainability reporting
Lack of data coverage is a major hurdle that can be overcome through the use of machine learning. Today, 80% of listed companies do not report required sustainability data. That means that, regardless of reliability issues, only 20% of publicly listed companies report comprehensive data on sustainability as a baseline. Many providers may then pile on partial or missing information, making it difficult to create consistent scores across peers and potentially skewing scores toward companies that disclose selectively by leaving out data on indicators for which they are behind. This is why Clarity AI leverages available company information and machine-learning algorithms to fill in the information gaps to give the fullest available picture.
Geographically, Europe has been leading the way with national regulations on climate change reporting for corporations, which crystallizes in the highest GHG reporting coverage among major world regions. Meanwhile, the US Securities and Exchange Commission is preparing a specific climate reporting regulation for 2022. The expectation is that reporting in North America will catch up to the rate in Europe within the next couple of years.
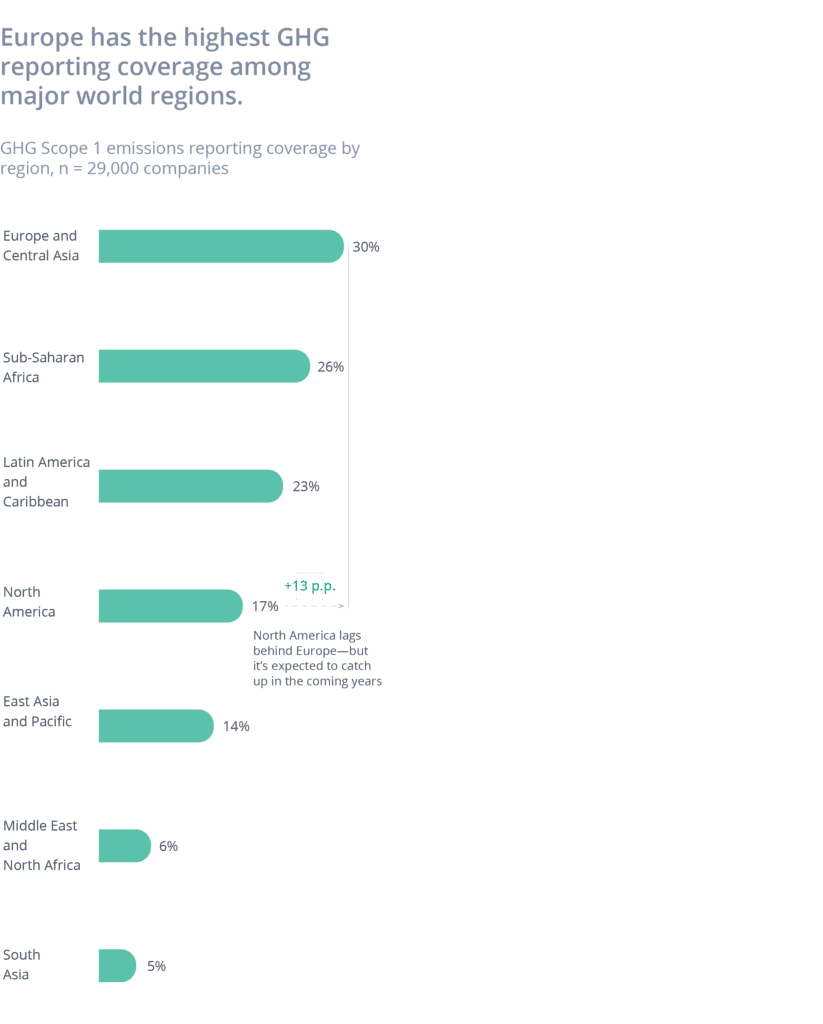
Clarity AI’s Estimation Models
One application of machine learning is our estimation models. The underlying principle of the models is to figure out how sustainability performance metrics can be derived from other corporate attributes. A wide range of both data sources and features (information about the organization) are used as input for the estimation models, including, for example:
- What industry are you in?
- What types of products and services do you sell?
- Are you a manufacturer?
- Where do you make your products? • Where do you sell your products?
- What are your labor costs?
- What are other environmental features that may be correlated with the metric of interest? (This depends on the metric.)
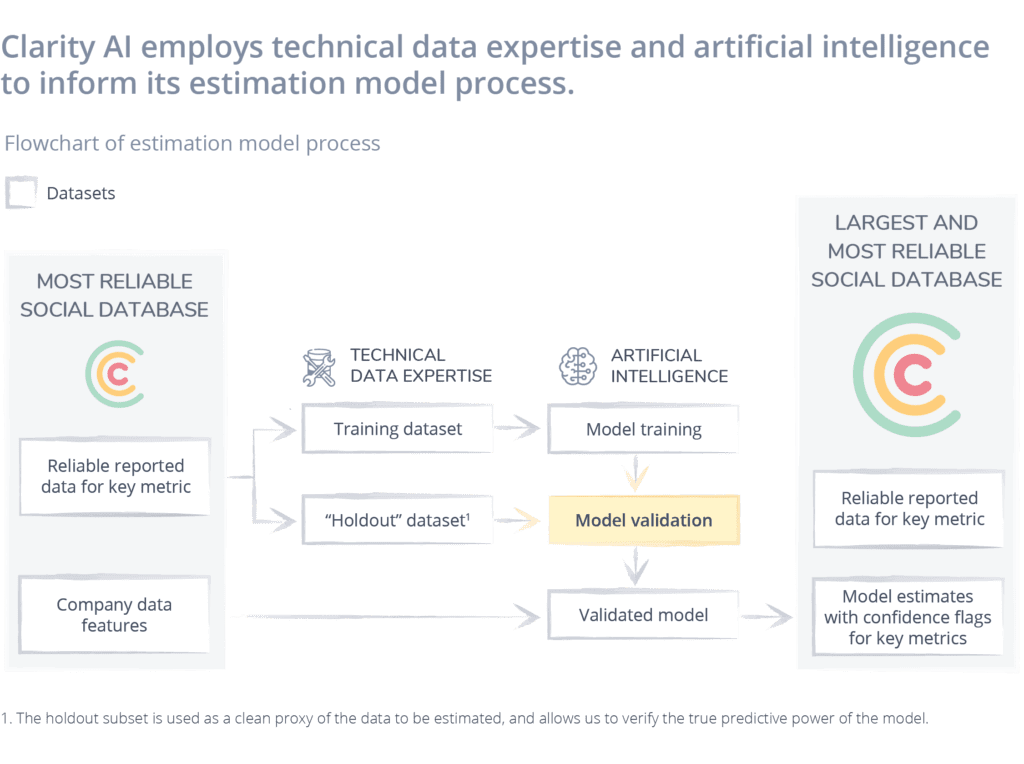
Key differentiators of Clarity AI’s methodology are the estimation of the intensity of the metric, the use of holdout data to test the predictive accuracy of the model, and accounting for both non-linear and interaction effects. These are crucial for estimating certain sustainability metrics as CO2 emissions.
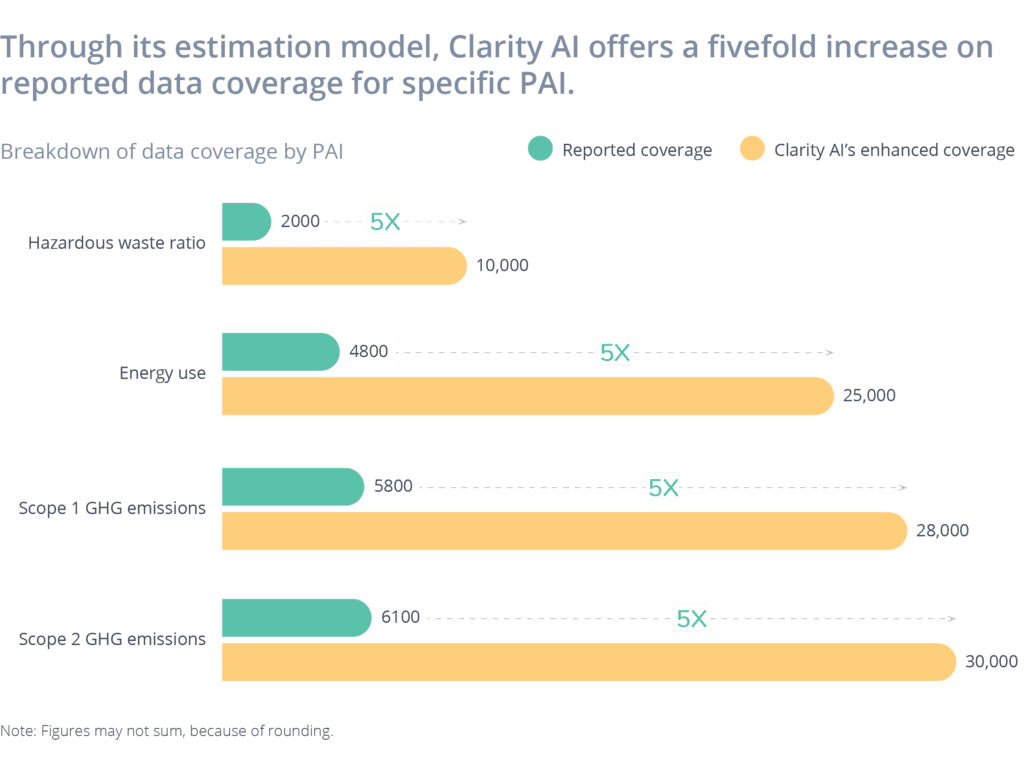